
The concept of predictive analytics has considerable longevity. It proves its usefulness more than ever, especially in this period of accelerated development of computers.
It is quite easy to look at modern technologies and think that all this appeared in the last 20-30 years. Simply, as in almost any field of activity, every innovation builds on what has already happened. In the case of technology and today’s topic – predictive analytics – this is a natural development that humans also have from birth.
Predictive analytics is a concept that encompasses various methods by which data can be used to make predictions. And the usefulness is almost unlimited, on countless levels and in many areas. The development of the field of artificial intelligence, or rather machine learning, added a significant increase in capabilities.
That way, if you have a complete set of data, you can get a prediction of how things will develop in the future. Can you predict what will happen? Not really, but it’s close.
Predictive analytics may sound like a new thing, but it’s actually been around for decades
Although we humans initially make predictions based on data, and this helps us adapt to our environment, this concept, as it is understood today, would not be possible without computers. OK, historically there is also the example of Henry Ford who optimized the production of automobiles, but at the time he didn’t have this umbrella to interpret the data. Returning to computers, from the first iterations in this field – in the 40s – the development of the field of data analysis also began. Computers had and still have this great advantage over humans: they can do many more calculations and perform repetitive operations without getting bored or tired.
With the development of the computer industry, the available data sets have also increased. It so happens that today, for example, weather forecasts are more accurate than ever (although they are still not 100% accurate) thanks to data analysis.
In addition to this significant social benefit, data analytics is used by many companies and has an incredible contribution to business, as the decisions that are made are more accurate and more relevant to the needs.
Statistics are an important element in this whole debate. Added to this is the variety of data, its volume (how many data points) and variability. Depending on the field, although this is the case in most cases, time must also be considered: how quickly the data can be collected and how quickly the first conclusions can be offered, after which decisions can be made. In some systems, this happens automatically through AI-based systems management. And last but not least, a huge contribution to the veracity of the data. It’s kind of natural that you can’t make decisions based on fake data.
While predictive analytics can be applied to most organizations, there are certain industries that will benefit the most from implementing these analytics.
Photo by Susa: Pexels/Lukas
In this sense, I spoke with Cosmin Tanase, manager of BI and data processing (Data Intelligence & Analytics), Cheil | Centtrade, about methods and how they can be used for optimization and decision making. In transport, for example, whether it is public or that which is intended for the transportation of goods from one place to another by various means. And public applications such as maps – Google Maps, Apple Maps or Waze – use such techniques to provide users with different routes to get to the desired location, suggest which is the fastest, and estimate how long the journey will take.
“The banking system is another example of an area where something similar is needed. When people approach banks for loans or savings, banks use predictive analytics to improve customer support, improve efficiency and detect fraudulent activity, customers who apply for a loan but are not trustworthy because predictive models can detect certain characteristics that are not ordinary pattern,” Kosmin explained. This analytics can also be used to maintain customer loyalty. And they are put to good use when it comes to preventing bank fraud, detecting spending habits, and alerting you when something is out of the ordinary.
Additionally, in healthcare systems, predictive analytics can help correctly diagnose or predict a patient’s treatment outcome based on his or her history and symptoms. “And in sales, it is important that the stores always have the goods that are bought most often. Otherwise, customers will choose them over competitors. At the same time, also in this area, thanks to the use of data analysis and access to large amounts of data, store employees can better understand consumer behavior and the motivation behind each product purchased,” he added. This is how decisions are made to increase traffic to a physical or online store, build loyalty, or create and consolidate a good customer journey.
I talked about Henry Ford earlier, and here it’s worth mentioning the manufacturing industry, factories where predictive analytics are used to optimize the flow of work, materials, and to identify potential problems and provide solutions for them in real time, automated.
Steps by which predictive analytics can be integrated into the business and limitations
There are some universal truths when it comes to predictive analytics. One is that such analyzes are only as good as the data and the processes involved in processing it. In addition, it is very important that the goal is clear and, implicitly, the process leading to it takes this into account. Also, understanding the goal (risk management, fraud detection, customer loyalty, etc.) is the first step for a business that wants to implement something like this. Data and models must then be considered according to the purpose they are intended to serve.
Next comes the selection and preparation of data for the modeling process, and this is one of the most time-consuming processes, since the data must be extracted, checked, adjusted. The model will use this data to “learn” from past events, and this process typically takes about 50-60% of the time.
It then divides the data set into two samples, one containing approximately 75-85% of the data on which the algorithm will be “trained” and the other sample being the one on which the algorithm will be tested for accuracy. .
Photo: Adam Nowakowski/Unsplash
Finally, there is the stage of implementing several machine learning algorithms and testing each of them based on the data set from which the model is trained. The performance indicators for each model are then compared, and the one with the best values is finally selected.
While a continuous process would be desirable, predictive analytics also has limitations. “For example, the accuracy and precision of predictive models is determined by the completeness and accuracy of the data used when the algorithms study the data set. Consequently, an incomplete data set (with missing values, absurd values, outliers) can lead to an incorrect predictive model. Or limitations caused by the knowledge of people on the project team. Depending on the complexity of the model needed to achieve the goal, for example, creating an algorithm to detect fraudulent activities, the team needs to be built on the basis of experience,” Kosmin explained.
To use this technique, it is important to remember that it is a duo: big data – predictive analytics, since the former is the basis of every model of predictive analytics. And for this reason, it is very important that the process of data collection, cleaning and preparation is carefully and properly implemented, as the process involves mathematical models applied to current data as well as historical data to predict future behavior.
You can use two types of data, both separately and simultaneously. On the one hand, there is historical data stored by the company, such as information about the company’s loyalty programs, which can be used to analyze the buying behavior of customers at the moment and subsequently predict which campaigns will be optimal to attract them. further.
“Depending on the industry the organization is in, any type of data can be collected and analyzed to get relevant information about how the company is doing (detection of sick patients in the healthcare system, consumer behavior in sales, content on social networks , which is preferred by each user, security fraud detection, etc.). Data types can be structured (historical transactions, production system errors, inventory information) or unstructured (interactions on social media channels such as tweets, posts, online reviews, emails, audio and video files).”
In the case of real-time data, the best example is the use of maps. There you cannot get a realistic estimate of the required time without constant monitoring and analysis. In general, the service area needs real-time analysis. Video streaming platforms or social networks also run on the same predictive analytics infrastructure.
Predictive analytics primarily provides competitive advantage through decisions made after transforming data sets that do not express much in their raw form, but which, when translated into predictive models, can yield accurate insights into target audiences. , needs and even decisions that can be made. The technology is already there, it’s just a solution When and as No if.
Article supported by Cheil Centtrade
Source: Hot News RO
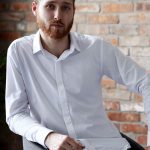
Robert is an experienced journalist who has been covering the automobile industry for over a decade. He has a deep understanding of the latest technologies and trends in the industry and is known for his thorough and in-depth reporting.